LoRA模型—探析(PyTorch)
LoRA
(Low-Rank Adaptation
) 是一种用于大型语言模型微调的高效技术。LoRA
旨在解决大语言模型微调时的计算资源和存储空间问题。在原始预训练模型中增加一个低秩矩阵作为旁路,只训练这个低秩矩阵,而冻结原模型参数。工作原理:在原模型权重矩阵
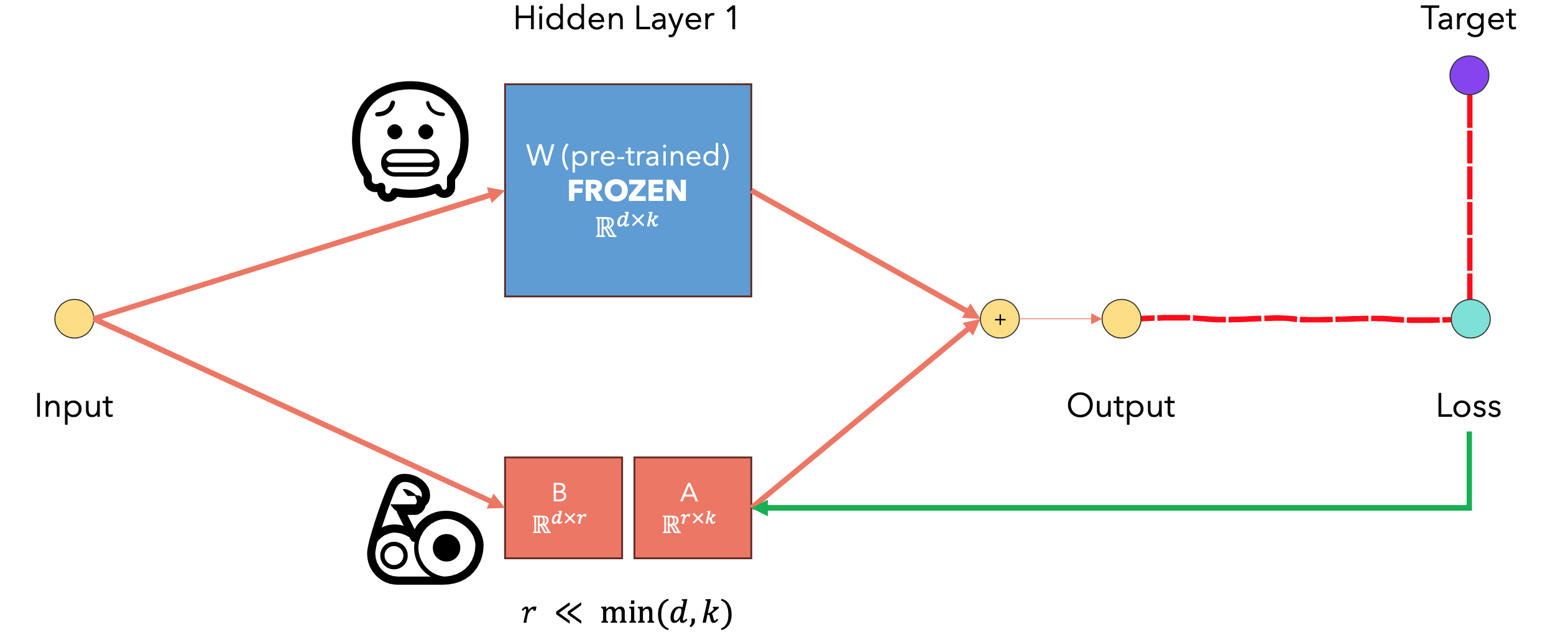
优点:大幅减少可训练参数数量,降低计算和存储开销;训练速度更快,使用内存更少。如果1%
。可以为不同任务训练多个LoRA
模块,便于切换。参数越少,存储要求越少。反向传播速度越快,因为我们不需要评估大多数参数的梯度。我们可以轻松地在两个不同的微调模型(一个用于SQL
生成,一个用于Javascript
代码生成)之间切换,只需更改LoRA
通过引入低秩矩阵作为可训练参数,有效解决了大模型微调的资源问题,为特定任务的模型适配提供了高效的解决方案。
预训练模型的
奇异值分解(SVD
):是一种重要的矩阵分解方法,接下来生成秩亏矩阵
1 | import torch |
结果输出为:
1 | tensor([[-1.0797, 0.5545, 0.8058, -0.7140, -0.1518, 1.0773, 2.3690, 0.8486, |
评估矩阵
1 | W_rank = np.linalg.matrix_rank(W) |
计算SVD
分解。
1 | # Perform SVD on W (W = UxSxV^T) |
给定相同的输入,使用原始
1 | # Generate random bias and input |
结果输出为:
1 | Original y using W: |
LoRA代码实现
我们将训练一个网络来对MNIST
数字进行分类,然后针对表现不佳的特定数字对网络进行微调。
1 | import torch |
结果输出为:
1 | Accuracy: 0.953 |
原始网络中有多少参数。
1 | # Print the size of the weights matrices of the network |
结果输出为:
1 | Layer 1: W: torch.Size([1000, 784]) + B: torch.Size([1000]) |
定义LoRA
参数化:PyTorch
参数化工作原理。
1 | class LoRAParametrization(nn.Module): |
将参数化添加到网络中。
1 | import torch.nn.utils.parametrize as parametrize |
结果输出为:
1 | Layer 1: W: torch.Size([1000, 784]) + B: torch.Size([1000]) + Lora_A: torch.Size([1, 784]) + Lora_B: torch.Size([1000, 1]) |
冻结原始网络的所有参数,仅微调LoRA
引入的参数。然后在数字9
上对模型进行微调,并且仅针对100
个批次。
1 | # Freeze the non-Lora parameters |
输出结果对比:
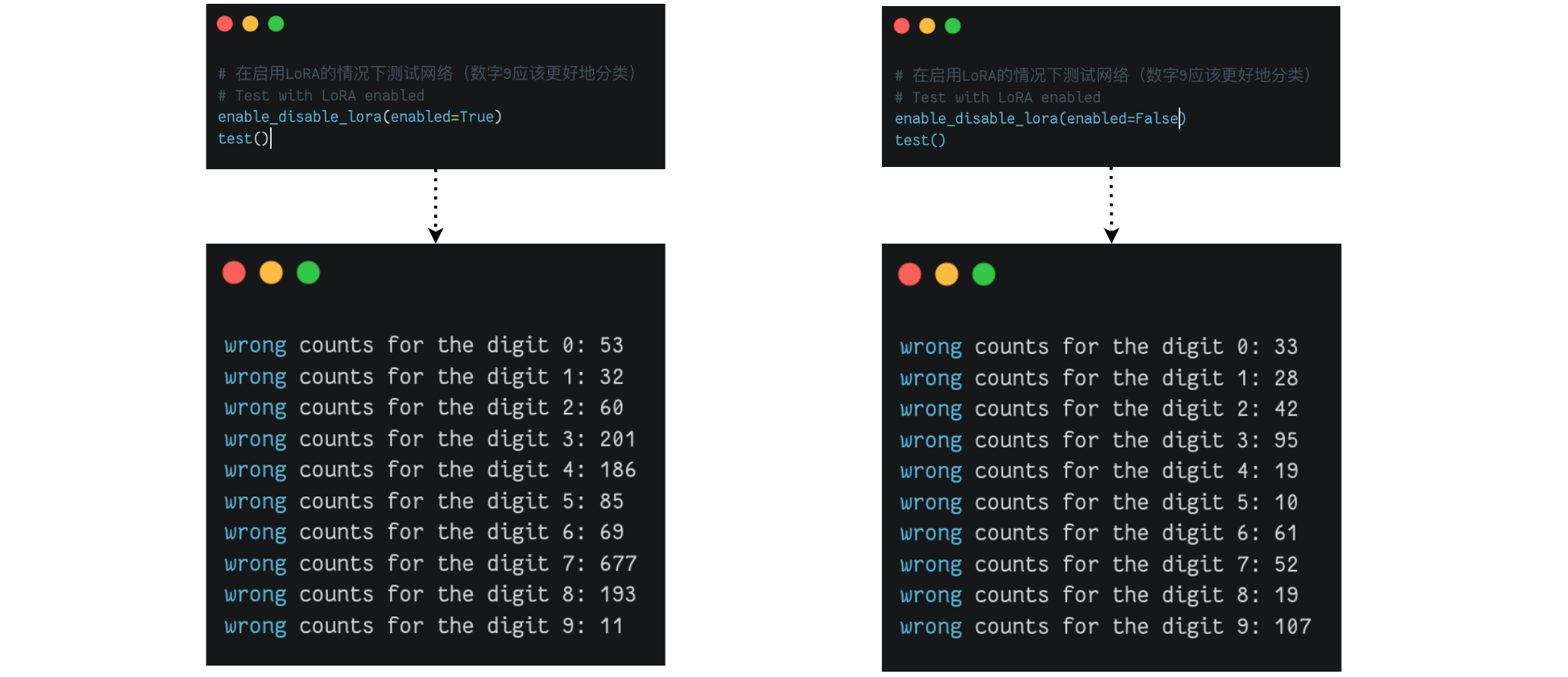